Correlation between New Parameters of Good Metabolic Control Obtained By a Blind Type of Continuous Glucose Monitoring Device and Laboratory Measured Glycated Hemoglobin A1c in Pediatric Patients on Multiple Daily Insulin Injection
* Gordana Bukara-Radujkovic;
-
* Gordana Bukara-Radujkovic: Department of Medicine, Pediatric Clinic, University Clinical Centre of the Republic of Srpska, Bosnia and Herzegovina.
-
Dec 22, 2022 |
-
Volume: 1 |
-
Issue: 2 |
-
Views: 515 |
-
Downloads: 613 |
Abstract
Introduction: Through retroactive analysis and statistical processing of the data obtained in the original research conducted in 2016, we want to establish which of the modern parameters of good metabolic control: Time In Range (TIR), Coefficient of Variation (CV) and Area Under the Curve below limit of 3.9 mmol/L (AUC below limit) had the greatest influence on the decrease of HbA1c, and whether it is in accordance with modern diagnostic recommendations for the treatment of type 1 diabetes mellitus in the pediatric population. The subjects in the original research were using a professional type of device for continuous monitoring, a blind type of device, and did not have immediate real-time insight into the glycemic values measured by the same, so they could not affect the results obtained.
Methods: Twenty four children with type 1 diabetes mellitus (10 boys and 14 girls), aged 5 years to 18 years old (average 12.0 ± 3.3), with an average duration of diabetes of 2.5 years, participated in the study. All subjects were treated with multiple daily insulin injections – MDI. According to the study protocol, the subjects were expected to perform control laboratory tests (HbA1c) and certain anthropometric measurements (height, weight, BMI) before the beginning of the examination. Each subject had to use a device for professional glucose monitoring once a month for seven days, three times over a three-month period. After each use of the device, the results were discussed with the subject, and if necessary, insulin doses were changed and advice was given on proper nutrition. After three months, HbA1c was again measured in the laboratory.
Statistical analysis: Initial HbA1c was 7.78 ± 1.17 (min.: 5.5%; max.: 10%). During the period of using the professional device for continuous glucose monitoring, there was a decrease in laboratory-measured HbA1c, and after three months the laboratory-measured average value was 7.34 ± 0.84 (min.: 5.60%; max.: 8.90%). The highest correlation coefficient and the only one that shows statistical significance in connection with laboratory measured HbA1c after three months is the time spent in the target range or TIR, and this correlation is negative. A linear regression model of the dependence of HbA1c on variables (TIR, CV, AUC below) was set up. The obtained R value of 0.820 (R2 = 0.673) tells us that the regression of HbA1c 82% is influenced by these three variables, the statistically significant of which is TIR (t = -5.411, p ≤ 0.01).
Conclusion: Subsequent statistical processing of the results showed that the decrease of HbA1c in the original research was most influenced by TIR, which coincides with modern methods and diagnostic recommendations. CV, as well as AUC did not show a direct impact on HbA1c, but did show an impact on TIR, which can be explained by the imperfections of the research itself. Through statistical analysis in this paper, we have shown that all parameters are related and that one depends on the other, so a TIR of 70% does not mean that there is no variability or hypoglycemic episodes, and therefore clinicians must observe all the data obtained using CGM devices in order to determine a good therapy.
Abbreviations
CGM: Continuous Glucose Monitoring; TIR: Time In Range; CV: Coefficient of Variation; AUC: Area Under the Curve; HbA1c: Hemoglobin A1c; BMI; Body Mass Index
Introduction
In recent years, the treatment of type 1 diabetes mellitus has progressed rapidly, combining the modern formulations of insulin preparations and medical devices, which patients use more and more often. In addition to the development of insulin delivery methods, through insulin pumps, the development of glucose monitoring devices is also significant, and special therapeutic effects are achieved by combining them [1]. This technological progress mostly affects the youngest patients group that is children and adolescents [2]. Furthermore, this technological progress influenced not only the improvement of metabolic control in this group of patients [3,4], but also changed the diagnostic classification of the disease itself and the parameters that describe good metabolic control. Sadly, statistic shows that a large number of patients in the world lack these medical tools in order to achieve optimal values of new parameters of good metabolic control. New parameters, that describe good metabolic control, are a direct product of using devices for Continuous Glucose Monitoring (CGM).
One of the most significant characteristic of the youngest population group of patients is that it is extremely difficult to assess when is the optimal time to introduce CGM into therapy [5], but it has been shown that adherence and acceptance of the device itself significantly influence the improvement of glycemic regulation, especially if they are introduced as early as possible in therapy. The large amount of data obtained by continuous glucose monitoring devices can confuse both patients and clinicians if they are superficially and incorrectly interpreted. Therefore, the 2017 ATTD consensus describes and explains in detail what values of certain parameters are considered as good metabolic control [6]. Old parameters, which have been used since the DCCT study [7], such as HbA1c, although still very present in daily clinical practice, due to the lack of CGM devices, are gradually being replaced by Time In Range (TIR), i.e. the time spent in the target range of glucose concentration. Values from 3.9 mmol/L (70 mg/dL) to 10.0 mmol/L (180 mg/dL) were taken as the limits of the range [8]. Many authors examined the relationship between HbA1c and TIR and all came to the conclusion that lower HbA1c means better TIR [9]. The cut-off value was 70% TIR, which is approximately equivalent to 7.0% HbA1c. In addition to TIR itself, a new parameter that describes good metabolic control is the Coefficient of Variation (CV). By convention, CV should be less than 36% for good metabolic control in the pediatric population [10].
The clinical and practical usefulness of these devices in therapy has been proven in a numerous studies in which the improvement of metabolic control during the period of using the CGM devices was demonstrated, and this improvement was reflected in the reduction of HbA1c, as well as hypoglycemic episodes [9]. Although most of these studies were conducted with participants using subcutaneous continuous insulin infusion through an insulin pump, there are also studies with people using multiple daily insulin injections, or MDIs, that came to the same conclusions [10]. Comparing these two groups of patients, with or without an insulin pump, it was shown that in patients without an insulin pump, a significantly greater decrease in HbA1c, i.e. an improvement in metabolic control, is achieved with the use of CGM [11].
The original research conducted in 2016 showed that there was a statistically significant decrease in HbA1c in children on MDI who used the device for continuous glucose monitoring for the first time [12]. Through retroactive analysis and statistical processing of the data obtained in this research, we want to establish which of the modern parameters of good metabolic control had the greatest influence on this decrease and whether it is in accordance with modern diagnostic recommendations for the treatment of type 1 diabetes mellitus in the pediatric population. It is important to note that all subjects in the original research were using a professional type of device for continuous monitoring, a blind type of device, and did not have immediate real-time insight into the glycemic values measured by the same, so they could not affect on the results obtained, which is advantage comparing to real-time glucose monitoring device. This fact was very important to the researcher because they wanted to get a true picture of glycemic control in this specific population group. A professional type of CGM device was the first choice for this study, as it is well-known that the pediatric population is generally very interested in technology and likes to interact with it, so the possibility of interaction and manipulation of results was very high if the real-time glucose monitoring device were used. However, these professional types of devices have their limitations and the most important one is the risk of hypoglycemia because there is no real-time glucose levels displayed to the user [13].
Methods
Twenty four children with type 1 diabetes mellitus (10 boys and 14 girls), aged 5 years to 18 years (average 12.0 ± 3.3), with an average duration of diabetes of 2.5 years, participated in the study. A criterion for inclusion in the study is a minimum duration of diabetes of one year. All subjects were treated with multiple daily insulin injections - MDI, or bolus-basal type of insulin therapy. The demographic characteristics of the group are described in (Table 1).
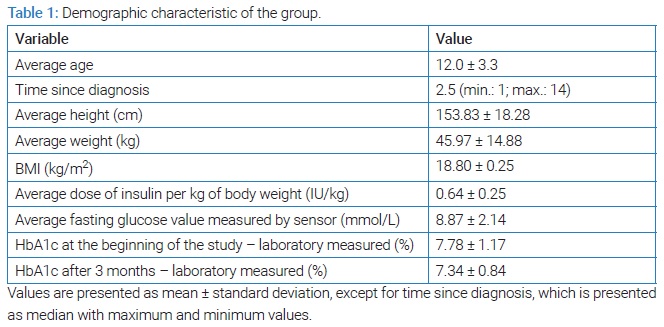
The study protocol was presented to the subjects and their parents/guardians before the start of the study. According to the protocol, the subjects were expected to perform control laboratory tests (HbA1c) and certain anthropometric measurements (height, weight, BMI) before the beginning of the examination. These tests did not include laboratory-measured fasting insulin due to established study criteria. One of the criteria is that all study participants had been diagnosed with diabetes for at least a year, so instead of fasting insulin, researcher took dosage of insulin per kg of body weight into consideration. However, fasting glucose concentration was measured in two ways: glucometers and a glucose sensor, and there were no significant differences between these two parameters during the study. Each subject had to use a device for professional glucose measurement once a month for seven days, three times over a three-month period. After each use of the device, the results were discussed with the subject, and if necessary, insulin doses were changed and advice was given on proper nutrition. Reviewing the results together with the research participant and counseling them after each use of the CGM device was a key part of this research, as it was shown that combining a professional type of CGM device and appropriate user education could lead to better glycemic control. After three months, HbA1c was again measured in the laboratory.
TIR limits are set from 3.9 mmol/L to 10.0 mmol/L, according to recommendations [6,8]. TIR were calculated automatically by software compatible with the professional type of device used in the study. The software records the minutes spent in TIR and calculate a percentage comparing them to the total time of device usage. CV was calculated using a mathematical formula for each subject individually, and the obtained values were further entered into the statistical analysis. The calculation formula represents the ratio of the standard deviation of all sensor measurements and the mean value of the glucose concentration measured by the sensor, expressed as a percentage [10]. As a parameter describing hypoglycemic episodes, the AUC below the limit was taken, and it represents the relationship between the duration of an individual hypoglycemic episode and all glucose concentration values measured by the sensor for the duration of the hypoglycemic episode. Also, this parameter was calculated by compatible software and the results were taken into further statistical analysis.
Considering that each subject used CGM for longer than 14 days in a period of three months and thus fulfilled the conditions for the calculation of the examined parameters [6], this essentially represents only 20.72% of the time spent using this device, and that is the first imperfection of this research. The second is a small sample, determined by the characteristics of the population on which the research was conducted.
All examined parameters in the statistical analysis were taken as mean values of all three measurements. This weighted mean value was subsequently obtained by a mathematical formula that used as a weight the minutes spent under CGM at each measurement. Descriptive statistics methods, correlation factors between parameters were used in the statistical analysis, and a linear regression model was set, where the dependent variable was HbA1c after three months of study, and the independent variables were: TIR, CV and AUC below the limit. All variables significant for statistical processing are numerical. Other parameters are not listed in this article, as we first want to examine the correlation between these mostly used parameters. Further analyzes should include more statistical parameters describing glucose variability.
Statistical Analysis
Initial HbA1c was 7.78 ± 1.17 (min.: 5.5%; max.: 10%). During the period of using the professional device for continuous glucose monitoring, there was a decrease in laboratory- measured HbA1c, and after three months the laboratory-measured average value was 7.34 ± 0.84 (min.: 5.60%; max.: 8.90%). As it shown in original research, this decrease is statistically significant, and the parameters describing that are ΔM = 0.45, t = 2.67, and Pbonf = 0.041 [12].
Statistical analysis presented in this article sought to determine which of the parameters of good glycemic control including: time spent in the target range (3.9 mmol/L to 10.0 mmol/L), coefficient of variation (≤ 36% was taken as a parameter of good metabolic control) [14], and AUC below the limit of 3.9 mmol/L (which should be as close as possible to 0), had the greatest impact on this decrease. (Table 2) lists the basic parameters of descriptive statistics for all three variables.
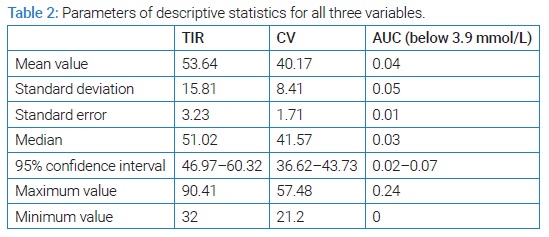
At first glance, the descriptive statistics do not favor good metabolic control of the examined group. The average TIR of 53.64% is not in agreement with the consensus recommendations of a minimum of 70.00% nor with the interpretations of the correlation between TIR and HbA1c [6,9]. Also, CV of 40.17% speaks of high glycemic variability in the group [10], and AUC below the limit of 0.04 indicates that hypoglycemic episodes occurred and with the obtained maximum AUC value of 0.24, we can assume that some of them were severe. However, the obtained decrease in laboratory-measured HbA1c tells us that there was an improvement during the study, and in further statistical processing we wanted to examine the correlation factors between the tested variables and laboratory-measured HbA1c after three months of using a professional system for continuous glucose monitoring. (Table 3) shows the Pearson correlation's coefficient of HbA1c and all examined variables with each other, with statistical significance. The highest correlation coefficient and the only one that shows statistical significance in connection with HbA1c is the time spent in the target range of TIR.
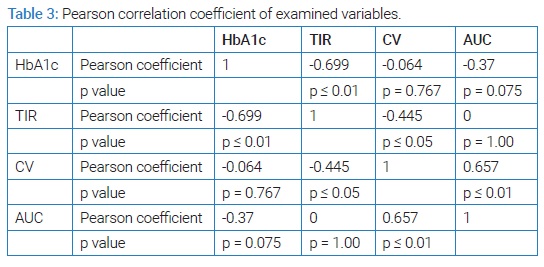
This correlation is negative, which favors the fact that the higher the TIR, the lower the HbA1c [9]. Other variables do not correlate statistically significantly with HbA1c, but have statistically significant correlations with each other. CV correlates negatively with TIR and positively with AUC. This supports the fact that higher TIR means less glycemic variability, and therefore lower CV, and that higher CV means more hypoglycemic episodes, i.e. higher AUC below the limit. Correlation factors lead us to the conclusion that: increasing TIR, we decrease HbA1c, CV and the number and severity of hypoglycemic episodes.
A linear regression model of the dependence of HbA1c on these three variables was set up. The obtained R value of 0.820 (R2 = 0.673) tells us that the regression of HbA1c 82% is influenced by these three variables, the most statistically significant of which is TIR (t = -5.411, p ≤ 0.01). The normal graph of the distribution of the observed and expected value of HbA1c in this model is shown in (Chart).
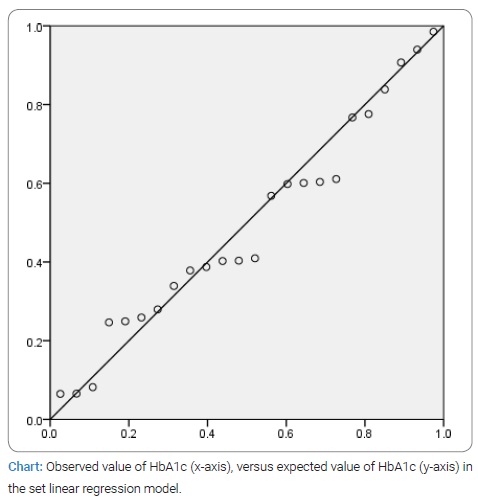
As already stated in the text, the imperfection of the study itself, which affects the statistical analysis, is the small sample caused by the character of the tested group. The analysis itself, however, gave statistically significant results and links between the examined variables [6–9,14–16].
Conclusion
Subsequent statistical processing of the results showed that the decrease of HbA1c in the original research was most influenced by TIR, which coincides with modern methods and diagnostic recommendations. CV, as well as AUC did not show a direct impact on HbA1c, but did show an impact on TIR, which can be explained by the imperfections of the research itself. The association of CV with AUC below the limit, which we took as a parameter for describing hypoglycemic episodes, is interesting. These two parameters are directly correlated, which means that a higher CV represents more hypoglycemic episodes. However, as a CV below 36% is considered good, we have to look at the AUC, or hypoglycemic episodes, even in these patients, because this does not mean that they are not present.
In addition to using a professional type of CGM device, it is necessary, in order for the user to benefit from it, to have a healthcare professional who would properly explain all the graphics and values obtained by this device. Proper nutrition and insulin dosing education of participants in our study led to better HbA1c, although there was a limitation of the study in terms of small sample size and short time spent on the CGM device, as mentioned above. Besides that, another limitation is that research was conducted in 2016, using the obsolete type of professional CGM device, and the results obtained were hard to make compatible with today's standards. Taking all that mentioned into consideration, the results, which show no statistical significance between CV, AUC under limit and HbA1c, were expected. However TIR shows statistical significance correlating with HbA1c and that is the true value of these analyses. Although HbA1c remains the standard of glycemic control, especially for poorer parts of the world who cannot afford any kind of CGM device, it may also represent an additional standard of control even for people who use these devices. A large discrepancy between the values may indicate that the device is faulty or that there is manipulation of the results by the user, or to confirm inaccuracy in measured HbA1c due to renal disease or hemoglobinophaty. A professional, blind type of device can help in this situation, and clinicians could benefit from it, especially in the pediatric and adolescent population.
Using a device for continuous glucose monitoring undoubtedly already gives good results in terms of therapeutic effect, but it also represents the future of diabetes therapy. The prevalence and wide use of these devices in therapy undoubtedly improves metabolic control. However, clinicians must be aware of all the data obtained using these devices, know how to interpret them and, of course, with the still present gold standard HbA1c, determine the best course of therapy for the patient. Through statistical analysis presented above, we have shown that all parameters are connected and that one depends on the other, so that a TIR of 70% does not mean that there is no variability or hypoglycemic episodes. The comprehensiveness of the image implies an overview of all parameters and their correct interpretation in order to avoid unwanted hypoglycemic episodes, which is particularly important in the pediatric population.
Conflict of Interest
The authors declare no potential conflicts of interest with respect to the research, authorship, and/or publication of this article. Informed consent was obtained for this publication.
References
- Soni A, Wright N, Agwu JC, Timmis A, Drew J, Kershaw M, et al. A practical approach to Continuous glucose monitoring (rtCGM) and freestyle libre systems (isCGM) in children and young people with type 1 diabetes. Diabetes Res Clin Pract. 2022;184:109196.
- Garg SK. The future of glucose monitoring. Diabetes Technol Ther. 2016;18 Suppl 2(Suppl 2):S2iv–22.
- Teo E, Hassan N, Tam W, Koh S. Effectiveness of continuous glucose monitoring in maintaining glycaemic control among people with type 1 diabetes mellitus: a systematic review of randomised controlled trials and meta-analysis. Diabetologia. 2022;65(4):604–619.
- Huhn F, Lange K, Jordening M, Ernst G. Real-world use of continuous glucose monitoring (CGM) systems among adolescents and young adults with type 1 diabetes: reduced burden, but little interest in data analysis. J Diabetes Sci Technol. 2022;19322968221081216.
- Lawson ML, Verbeeten KC, Courtney JM, Bradley BJ, McAssey K, Clarson C, et al. Timing of CGM initiation in pediatric diabetes: the CGM time trial. Pediatr Diabetes. 2021;22(2):279–287.
- Danne T, Nimri R, Battelino T, Bergenstal RM, Close KL, DeVries JH, et al. International consensus on use of continuous glucose monitoring. Diabetes care. 2017;40(12):1631–1640.
- Diabetes control and complications trial (DCCT): results of feasibility study. The DCCT research group. Diabetes Care. 1987;10(1):1–19.
- Battelino T, Danne T, Bergenstal RM, Amiel SA, Beck R, Biester T, et al. Clinical targets for continuous glucose monitoring data interpretation: recommendations from the international consensus on time in range. Diabetes Care. 2019;42(8):1593–1603.
- Vigersky R, McMahnon C. The relationship of hemoglobin A1C to time-in-range in patients with diabetes. Diabetes Technol Ther. 2019;21(2):81–85.
- Dovc K, Cargnelutti K, Sturm A, Selb J, Bratina N, Battelino T. Continuous glucose monitoring use and glucose variability in pre-school children with type 1 diabetes. Diabetes Res Clin Pract. 2019;147:76–80.
- Garg Sk. Role of continuous glucose monitoring in patients with diabetes using multiple daily insulin injections. Infusystems USA. 2009;6(2):9–14.
- Gordana RB, Vesna M, Sinisa L. The effects of three-month-long continuous glucose monitoring in children with type 1 diabetes on multiple daily insulin injections. Vojnosanit Prergl. 2018;75(12):1209–1215.
- Jensen MH, Vestergaard P, Hirsch IB, Hejlesen O. Use of personal continuous glucose monitoring device is associated with reduced risk of hypoglycemia in a 16-week clinical trial of people with type 1 diabetes using continuous subcutaneous insulin infusion. J Diabetes Sci Technol. 2022;16(1):106-112.
- Dovc K, Van Name M, Bizjan BJ, Rusak E, Piona C, Yesiltepe-Mutlu G, et al. Continuous glucose monitoring use and glucose variability in very young children with type 1 diabetes (VibRate): a multinational prospective observational real-world cohort study. Diabetes Obes metab. 2022;24(3):564–569.
- Ahn D, Pettus J, Edelman S. Unblinded CGM should replace blinded CGM in the clinical management of diabetes. J Diabetes Sci Technol 2016;10(3):793–798.
- Johnson SR, Holmes-Walker DJ, Chee M, Earnest A, Jones TW, ADDN Study Group, et al. Universal subsidized continuous glucose monitoring funding for young people with type 1 diabetes: uptake and outcomes over 2 years, a population-based study. Diabetes Care. 2022;45(2):391–397.
Keywords
Type 1 diabetes mellitus; HbA1c test; Hypoglycemic episodes; Insulin; Glycemic value
Cite this article
Bukara-Radujkovic G. Correlation between new parameters of good metabolic control obtained by a blind type of continuous glucose monitoring device and laboratory measured glycated hemoglobin A1c in pediatric patients on multiple daily insulin injection. Glob J Pedia. 2022;1(2):1–6.
Copyright
© 2022 Gordana Bukara-Radujkovic. This is an open access article distributed under the terms of the Creative Commons Attribution 4.0 International License (CC BY-4.0).